Europe Aims to Reach 75% AI Adoption by 2030. Here's How Intelligent Engineering Can Help. The opportunities remain wide open, yet enterprises are moving much more slowly than those in other regions of the world.
By Mahesh Raja Edited by Jason Fell
Opinions expressed by Entrepreneur contributors are their own.
You're reading Entrepreneur Europe, an international franchise of Entrepreneur Media.
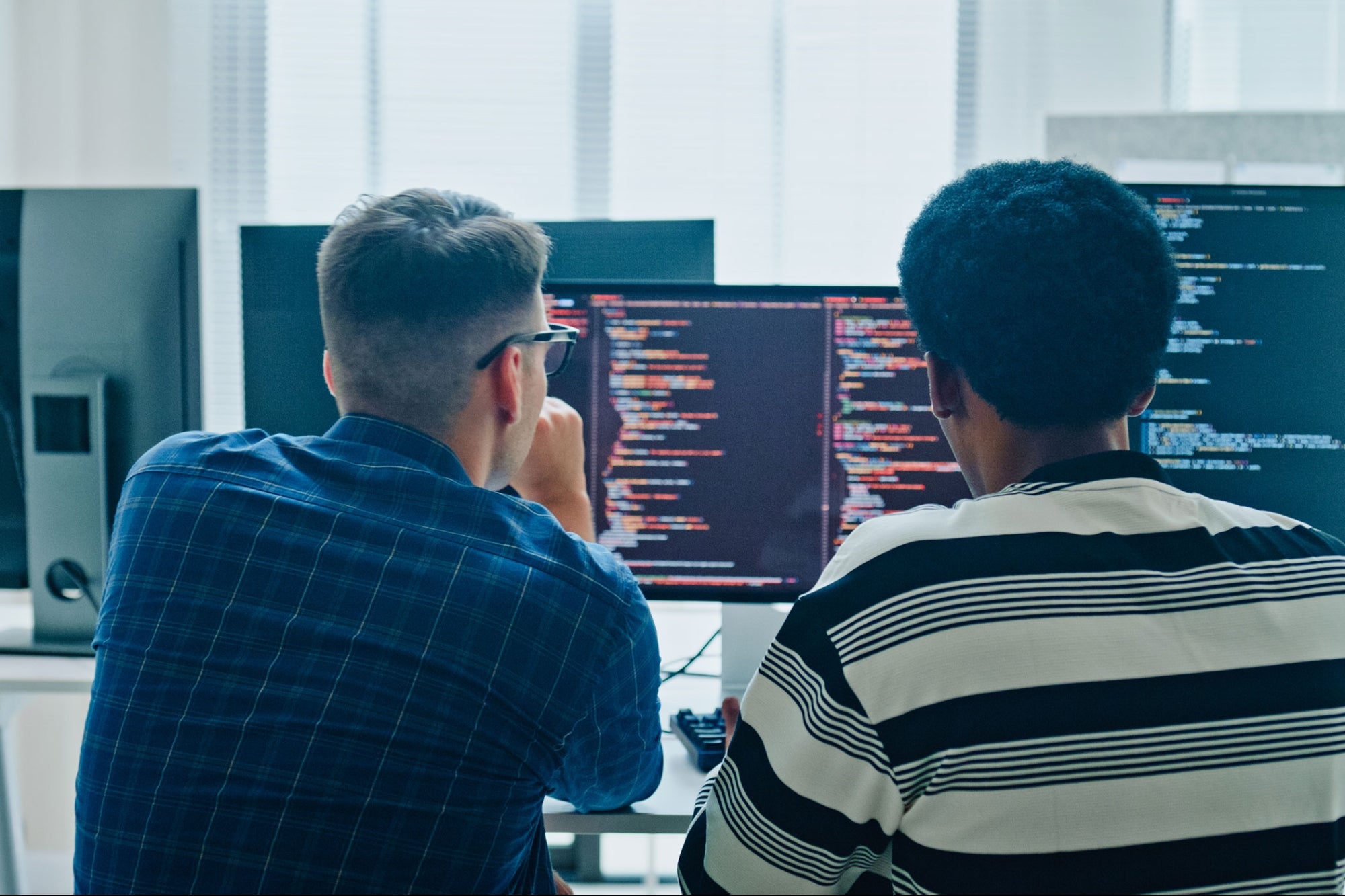
This February the global artificial intelligence (AI) community looked to Europe for one of the most anticipated AI events of the year. The AI Action Summit, held on February 10 to 11 in Paris, saw more than 1,000 participants, including several dozen heads of state, explore the latest breakthroughs and opportunities for this technology.
Hot off the heels of these event proceedings, it's clear that the industry has gone through a seismic shift. The previous summits, including the UK AI Safety Summit and AI Seoul Summit, focused on risks and safeguards, whereas the Paris summit looked at the potential of the technology.
For the region in particular, this represents a significant shift in attitudes that have previously focused on heavy regulations that many argue have placed EU's innovation at risk.
This is likely to have been spurred on by Mario Draghi's September 2024 report into competitiveness, which noted that AI will be the "crucial driver of productivity'' and that those who embrace the opportunity will fast-track growth. For example, GenAI could add $575.1 billion to Europe's economy by 2030, according to McKinsey.
In this critical moment for Europe, AI must move from a conceptual technology into one that can be rapidly adopted and put to action. While a combination of factors have contributed to slower adoption rates in the region, one barrier to adoption comes in the form of software efficiency.
Here, Intelligent Engineering, which leverages the power of data and intelligence to improve engineering productivity, can help to overcome software development inefficiencies to reach higher adoption targets and help founders and CEOs embrace a new era of AI adoption.
Helping reach first-mover advantage
For companies to realize the benefits of AI, its core that the pace in which the technology is being adopted and applied is accelerated.
For one, rates are currently well below targets, with today only 11% of EU companies are currently adopting AI, a fraction of the target 75% the bloc aims to reach by 2030.
As with any innovation, there is a significant first-mover advantage to be had. The technology is still in its early stages, and most productivity potential has yet to be captured, so the opportunities remain wide open. Yet enterprises are moving much more slowly than those in other regions of the world.
To close this gap, velocity is an important metric for enterprises in order to improve AI competitiveness.
However, several converging megatrends need to be addressed before this can be achieved. For example, the evolution of cloud computing has had a phenomenal impact on the way that software development processes operate. Further, regions like the U.S. often have greater access to hyperscale marketplaces that further boost agility and therefore competitiveness.
The need to deploy more software within shorter periods is also placing unprecedented pressure on the performance of engineering teams.
Together these trends mean the need for high-performing engineering teams is at a peak, however, making improvements has never been more challenging. Today, more than ever before, a data-driven approach is needed as a framework to continuously improve upon software engineering velocity.
Intelligent Engineering starts with data
Improving the velocity of software release cycles and adopting an intelligent approach to software engineering starts with data. Complex development pipelines mean that the root cause of performance issues aren't always easily apparent. An end-to-end data analysis means that data-driven actions can be taken to effectively improve overall rates of velocity and realize software innovations.
This is particularly important when we consider how the rise of cloud computing and citizen developers have caused a fragmentation of the development environment. For instance, every team often has a DevOps tool in place to manage workflows. Yet these metrics are most often being measured in isolation.
This means that the KPIs that a team is working towards don't necessarily have any impact on the actual velocity of software release cycles, nor do they consider how the teams' deliverables interact with other stages in the pipeline.
Data needs to be collected and measured from each constituent part and analyzed as a whole. This moves us away from a siloed approach to productivity management to instead offer complete visibility.
This type of detailed analysis ensures that the root causes of the problem can be identified and solved swiftly, applying help to the exact points that are needed across the development process.
Speed and quality drive improvement decisions
When it comes to AI adoption, European organizations lag behind their U.S. counterparts by 45% to 70%.
However, most of GenAI's economic potential lies in these early gains. This is why Europe stands to benefit most from the next stage of Intelligent Engineering that focuses on speed and quality.
By working with the performance metrics from the data analytics, enterprises are able to take appropriate action. At the same time, the challenge of knowing which KPIs to apply to which teams remain.
For this reason, an Intelligent Engineering approach uses speed and quality as the two categories needed to guide decisions.
It's likely that some teams are already performing well in one or both of these categories. Identifying the lowest performers and making changes to these teams first and foremost will have a greater and more immediate impact, in line with the Intelligent Engineering approach.
Continuous improvement mindset
Once the most urgent roadblocks and biggest sources of waste have been identified and tackled, a core part of Intelligent Engineering is understanding that this is a continuous process. After an action has been taken, the impact of pulling this lever needs to be traced and monitored to understand how it has impacted the overall release cycle.
For example, if 'requirements stability' has been identified as a source of waste affecting speed, a logical action to improve the process maturity and requirements management would be to apply a GenAI tool like CoPilot.
When we have complete visibility of the development cycle, we can not only identify the root cause of poor velocity, but can take appropriate action and get an exact measure on the impact. This approach recognizes that it is not a 'once and done' type exercise. Instead, the systems harness the incredible power of analytics to continuously monitor and eliminate waste as an active process.
Why this matters now
As seen at the AI Action Summit 2025, the global AI industry is now ready to move in the action-driven phase of adoption. For Europe, working to adopt this technology at a faster pace is key to driving EU competitiveness on the global stage by unlocking an important first mover advantage.
The Intelligent Engineering approach outlined here offers a way to boost software engineering velocity and bring AI solutions to European organizations.
Process, automation and AI are all levers to do this; however, these must also be implemented systematically. Intelligent Engineering eliminates waste, boosts productivity and tackles the complex and fragmented reality of modern software engineering.